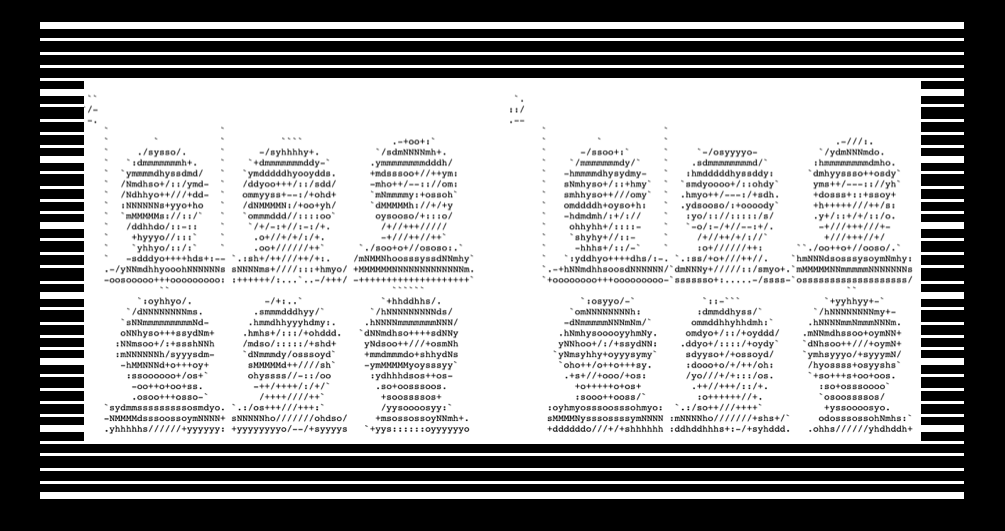
Investigating algorithmic bias in criminal justice
by Mack Willett | April 19, 2019
When Eric Loomis was sentenced to six years in a Wisconsin prison, he didn’t know why. What he did know was that he had been detained in connection to a drive-by shooting in La Crosse. The car he was driving had been the getaway vehicle. He even pleaded guilty to two of the less severe charges: “attempting to flee a traffic officer and operating a motor vehicle without the owner’s consent.” He just couldn’t understand why the judge had given him six years. More importantly, he did not know the factors and decisions which had dictated the sentence. This was because they had been weighed up and assessed by a computer.
The judge who adjudicated the trial had used data provided by COMPAS (Correctional Offender Management Profiling for Alternative Sanctions), a risk assessment algorithm which predicts the risk of a defendant committing another crime. Based on an interview with the defendant, a 137-item questionnaire, and their criminal record, the algorithm gives defendants a risk score from 1-10. It’s used across the US in crime prediction, prison treatment, and inmate supervision. In Loomis’ case, it was used to determine an appropriate sentence. The algorithm, written by software company Equivant (recently rebranded from Northpoint), is supposed to provide supplementary data to inform a judge’s decision; a high-risk score usually means a longer or harsher sentence. In theory, the software appears to be the perfect tool for delivering fair and appropriate justice, but in practice the algorithm proves much more complicated.
One year after his conviction, Loomis and his lawyer brought a case to the Wisconsin Court of Appeals. Loomis contended that the use of the algorithm violated his right to due process because of its lack of transparency. Although the court could view the risk score and the inputs which affected it, Loomis argued that Equivant was constitutionally required to disclose the algorithm’s code. After all, not even the judge knew which decisions the software had been programmed to make. The appeal went up to the Wisconsin Supreme Court, which ultimately ruled against Loomis, but called for caution in the use of predictive algorithms like COMPAS.
Loomis’ case isn’t the only time COMPAS has come under scrutiny. In 2017, Glenn Rodríguez, an inmate at the Eastern Correctional Facility in upstate New York, was denied parole despite an almost spotless rehabilitation record. Like Loomis, Rodriguez was bewildered; his COMPAS score was to blame. Unlike Loomis, however, he realised that the algorithm’s lack of transparency was not the only issue. Rodríguez was able to review his questionnaire, which provided inputs to the software, and found a mistake: one of the correctional officers who had been filling out the 137-item form had given an inaccurate answer to question nineteen. He talked to other inmates with similar scores to him in an effort to comprehend the significance of that single question, and, after some searching, found someone with an almost identical questionnaire. All their answers were the identical except for question nineteen, and yet their scores were entirely different. He brought his findings before the parole board, contending that if an input had been wrong, his final risk score couldn’t possibly be right. But Rodríguez ran into the same obstacles as Loomis: without knowing how inputs were weighted in the algorithm’s code, he wasn’t able to prove how important the error was, or indeed persuade anyone to correct it. Sat in front of the parole board, he was forced to argue his case without being able to challenge the score or how it was calculated. Rodríguez was granted parole in May 2017, but would never be permitted to dispute the troubling issues of his COMPAS risk score.
Rodriguez and Loomis’ cases illustrate the extent to which minor details and opaque systems can impact defendants’ cases and alter people’s lives. Taken in isolation, these cases may seem like minor anomalies, but it’s when the issue of transparency intersects with racial bias that the ramifications of these kinds of errors really hit home. Programs like COMPAS operate in environments where racial bias is present at almost every juncture. The criminal justice system in the US was designed to preserve racial order in the Jim Crow years of the nineteenth century. Then laws enforcing racial segregation were implemented, and today’s justice system retains much of its function in this regard. Statistically, the racial disparity in police shootings of black people cannot be explained by higher crime rates in majority-black communities. Ava Duvernay’s documentary, 13th, notes that “police violence isn’t the problem in and of itself. It’s a reflection of a much larger brutal system of racial and social control, known as mass incarceration, which authorises this kind of violence”. Black people are overrepresented in the prison population. When black men and white men commit the same crime, black men receive an average sentencing of almost twenty-percent longer. Even at a judicial level, politically motivated legislation and court processes such as the encouragement of plea bargaining are all specifically prejudiced against the US’s African-American population. Structures of systemic racial discrimination are the context in which COMPAS operates, a context which significantly raises the stakes in any discussions of institutional transparency in the United States.
In 2016, ProPublica, a Pulitzer Prize-winning non-profit newsroom focused on civil rights journalism, ran an investigation into COMPAS. It raised troubling conclusions: problems with algorithmic justice actually ran far deeper than previous isolated incidents suggested. ProPublica found the software to be heavily biased against black people. Juxtaposing a list of convicts against one another – one black, the other white or Latino – black people were consistently given what seemed like inflated risk scores despite input factors suggesting the opposite. In one case, a black woman with four juvenile misdemeanours was given a score of eight. By contrast, a white man with two armed robberies and one attempted armed robbery was given a score of just three. Even when controlling for prior crimes, recidivism (likelihood of reoffending), age, and gender, black defendants were forty-five-percent more likely to be assigned higher risk scores than their white counterparts. Equivant rejected ProPublica’s findings. Still, the media spotlight grew, with several news outlets running a sequence of follow-up investigations. The Washington Post’s analysis centred around definitions of fairness and disputed elements of ProPublica’s methodology, but ultimately reiterated concerns of transparency. Despite the media storm, Equivant still rejected calls to release the algorithm’s code for investigation. Just like Loomis and Rodriguez, ProPublica and other media outlets had run into the same transparency problem; unable to inspect the algorithm and relying only on input and output data, the investigations could not conclusively prove any racial bias in the system.
It is well-known that algorithms are far from objective. They have the potential to be just as flawed as human decision-making: many stages in COMPAS’ process could allow for bias to seep in. Maybe the system is trained on data which reflects the police’s own racial discrimination. Perhaps the data is too narrow and fails to encapsulate a diverse spectrum of criminals and citizens. Most probably, certain input variables are weighted differently to others, variables that can skew final scores. In any case, what is alarming about the Equivant case is not only the possibility of racial bias, but also the glaring issue of transparency. Since Equivant is a for-profit organisation, the COMPAS code is protected from inspection by trade secret laws. Software like this is usually protected because code is often not patentable, like an abstract idea or mathematical formula. Protecting it can incentivise new intellectual creations. However, in the case of COMPAS, trade secret laws permit private companies to withhold information, not only from competitors or patent-thieves, but also from the likes of Rodríguez and Loomis, individual defendants with sentences on the line. Accessing information about how these technologies work can be critical to a defendant’s case, but it involves a seemingly unsolvable legal contradiction between freedom of information and trade secrecy. In the context US mass incarceration, it is a dispute which urgently needs a resolution.
Already ingrained in America’s institutional fabric, the global diffusion of algorithm tech means that these same issues are steadily spreading. Elsewhere in the global justice system, algorithms, machine learning, and prediction software are being implemented with equally concerning results. Take PredPol, a crime-prediction software used in the UK and the US, which calculates high-risk areas and allocates police patrols accordingly. Like COMPAS, PredPol is privately owned, generates between $5-6 million a year, and is – of course – incredibly secretive. It took years of public pressure for PredPol to finally release a general description of their algorithm in 2016. It was immediately investigated by the Human Rights Data Analysis Group and the findings were predictably gloomy. The algorithm was found to exacerbate past racially biased policing practices: PredPol would home in on areas overrepresented in previous arrest data (predominantly black neighbourhoods), increase police patrols in those sectors, and then use the corresponding spike in reported crime to validate its predictions. An unsettling feedback loop. It was argued that the bias ultimately came from the training data. Nevertheless, it is alarming to think that were it not for public pressure on Predpol to release a ‘transparent’ description of their software, their flawed system would still be in use today.
Bias and transparency are the key concerns regarding the use of algorithms in the criminal justice system. It is no wonder that organisations such as the American Civil Liberties Union (ACLU) are homing in on algorithmic bias as a pivotal area of civil rights investigation. It is somewhat ironic that a central issue for an institution which prizes transparency is transparency itself; it reflects the duplicitous nature of a criminal justice system which we already know to be riddled with injustices. Without consistent and rigorous investigation, algorithmic decision-making could become just another step in a legal process already rigged against racial and ethnic minorities. If we fail to act, algorithms such as COMPAS and PredPol will transpose systemic oppression into the digital era: an insidious, sanitised, digital version of age-old racial injustice. Think of the alternative – these are useful tools which could mitigate racial bias by eliminating human error. Whilst problems at the structural level might remain, algorithms implemented with appropriate caution, cooperation, and transparency could be a huge step towards change in how justice is delivered. Issues of algorithmic bias and transparency are not limited to the US; there are equivalent problems in UK policing and our own sentencing system. Such algorithms reinforce the idea that, as digitization becomes global, the accompanying problems become global too. The world is headed towards a tipping point. The sooner we face up to the insidious consequences of biased and opaque algorithms, the better.∎
Words by Mack Willett. Artwork by Mack Willett.